A note from the guest editor:
Doug Cavener’s incredible progress has been possible because Wolcott-Rallison syndrome is caused by a single gene mutation that affects a member of a well-known and well-studied protein kinase family and consequently, the mutation causing the syndrome could be found and studied using current state-of-the-art genomic analyses. However, the “target genes” for most human diseases are far more complex and far more difficult to find and study, simply by comparing the genomes of healthy and diseased individuals. In many cases, mutations in any of several genes can have the same or similar effects; or mutations can occur in genes that regulate the expression of many other genes; or they can occur in areas of the DNA that do not form proteins but regulate the expression of proteins; or they can occur in genes that control tissue-specific expression; etc. Consequently, current state-of-the-art genomic analyses—even when coupled with advanced genome-wide association studies (GWAS)— typically identify 100 or more potential target genes! Researchers at universities and pharmaceutical companies then begin the hard, time-consuming, and costly process of detailed laboratory investigations into each potential target. Yu Zhang (statistics) developed a novel statistical approach that incorporates genomic data, GWAS, and tissue-specific gene expression data (the epigenome) to pinpoint appropriate targets. But how would he know if his new analytical method worked? Using publicly available data sets, Zhang narrowed the number of targets to a handful for each of three complex diseases. He then worked with the college’s Office for Innovation and the University’s Office of Technology Management to protect his invention and to form a collaboration with GlaxoSmithKline to validate his analytical method with lab-bench data. The college recently awarded Zhang a Lab Bench to Commercialization grant to further develop and validate his statistical methods and to produce user-friendly software that should accelerate the production of new life-saving pharmaceutical products. —Andy Stephenson
Sequence to Solution
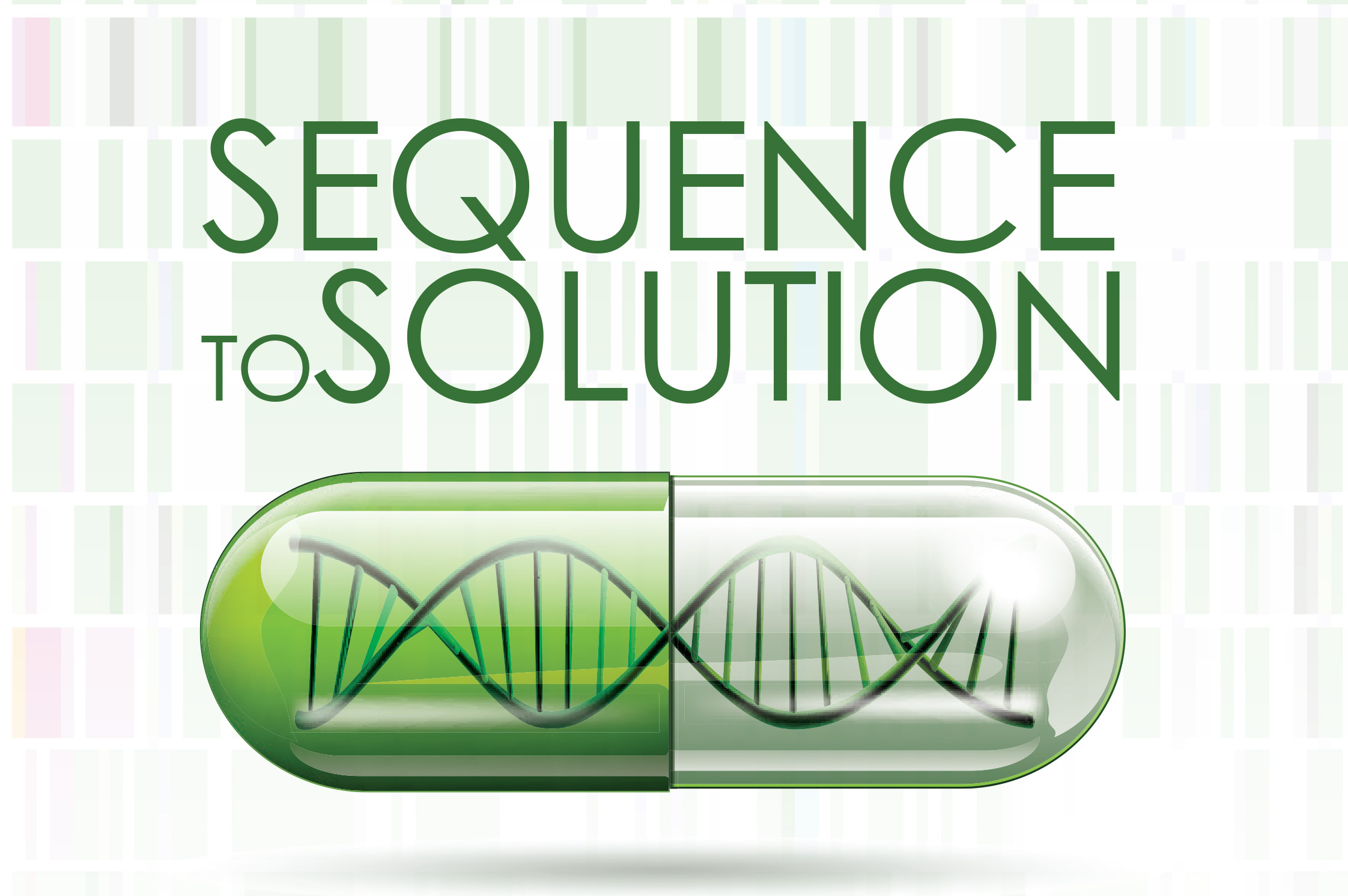
Your medical treatment may one day be just as customizable as your car. While drug therapy may not come in quite as many colors, customized medical decisions based on an individual’s genetic code may now be a slightly larger speck on the horizon thanks to new ways of consolidating genetic information.
A customized medical approach, often termed personalized or precision medicine, could allow medical professionals to predict how a patient would respond to different drugs and thus select the most effective drug and dosage with the fewest negative side effects for that individual. Expectations for personalized medicine have soared as new technology makes sequencing an individual’s genome faster and more affordable. But connecting a disease to specific alterations in a person’s DNA takes more than just sequencing the ATCG “alphabet” of an individual’s genetic code. Beyond learning the ABCs of a sequence, scientists must understand the significance of the “words,” how they relate to each other in a genetic “sentence,” and how “typos” cause disease.
A wave of research studies in recent years has focused on identifying mutations—changes to the ATCG code—associated with the risk of having particular diseases. Researchers are working to understand genes and other elements of the genome where these mutations occur, which can provide clues about the underlying biology of the disease and help identify targets for drug treatment. But it is rarely one mutation that causes a disease, and these genome-wide association studies (GWAS) might identify a hundred or more mutations associated with risk for a particular disease. These mutations could ultimately lead to misregulation or dysfunction of almost as many proteins, which in turn could be considered as targets for drug treatment.
“We have so much information from these studies,” said Penn State statistician Yu Zhang, “but we are ultimately left with the same question: What can these mutations tell us?”
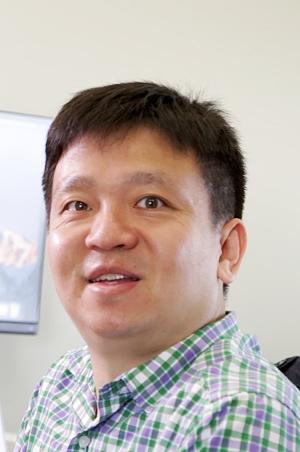
Zhang, an associate professor of statistics, has spent the last few years developing a statistical method to synthesize the abundance of data generated from recent studies. His method, called the Integrative and Discriminative Epigenome Annotation System (IDEAS), breaks the genome into segments of the sequence associated with different regulatory units that guide how and when the genetic code is deciphered. Researchers can then make notes about the function of elements within the segments, make connections with disease, share the information with other researchers, and ultimately help narrow down the list of targets for future drug therapies.
Too much information
Researchers have used GWAS to find disease associated elements of the genome since 2005. These studies identify specific mutations that occur with higher frequency in individuals with a particular disease, and mutations that are statistically associated with risk for the disease then become candidates for future biological study. Although the method cannot pinpoint which mutations actually cause the disease, it has still impacted the world of medicine. For example, a single mutation originally identified by GWAS was later shown to be strongly associated with how patients respond to drug treatment for the hepatitis C virus. Theoretically, this could allow physicians to customize medical decisions about using this drug based on whether a patient has the specific mutation; this sort of customized decision making is the basis of personalized medicine.
Like the mutation associated with response to hepatitis C treatment, many mutations identified by GWAS require further study to confirm their actual impact or function within the body. By the end of 2013, the results from almost 2,000 GWAS had been published, leaving tens of thousands of mutations associated with disease risk open for investigation.
“We developed the IDEAS method as one way to deal with all this data,” said Zhang. “IDEAS integrates large quantities of genetic and epigenetic data and predicts 20 to 30 distinct regulatory units of DNA sequences that might be substantially enriched in mutations involved in causing the disease.”
To identify segments of the genome that could contain disease-related mutations, IDEAS combines data about mutations discovered by GWAS with data from other studies of the biology and function of the genome. This includes information from studies of the modifications made to the proteins that package DNA inside the cell—modifications that can influence the way genes are expressed without altering the genetic code. These “epigenetic” modifications leave tags called chromatin marks that act as signposts for the changes. A study might investigate tags along the genome from one or two types of these chromatin marks, and in some cases signals from more than a dozen types of marks have been compiled. IDEAS looks for patterns in these chromatin marks to predict which elements in the genome might contain a disease-causing mutation.
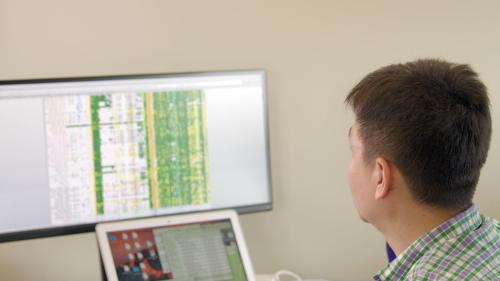
IDEAS condenses complex genetic data from different types of studies into genetic regions called epigenetic states, which carry information about the function of the segments. By doing so, it simplifies the data, reducing the number of dimensions and cleaning up the statistical “noise” that is inherent in the data and can cloud interpretation. This makes the data easier to visualize, analyze, and interpret.
Beyond genes
Researchers have used other statistical models to try to synthesize all of the genetic data being produced today, but most only focus on data from one cell type at a time. Although an individual’s cells all share the same genome, the genes are activated in different ways in different cell types— for example, to express proteins that make a lung cell or a liver cell unique. These differences result from a variety of regulatory elements in the genome that turn genes on and off or ramp up expression of certain genes under specific circumstances.
“Usually researchers look at the genes,” said Zhang, “but now we know that genes are simply the workers in the cell. We’re trying to find out where the managers of the cell—these regulatory elements—are located and identify the genes that they regulate.”
Genes were the first functional element of the genome that scientists understood, and many diseases are caused by mutations in genes. Genes code for proteins, which are the main structural and functional units of the cell, but genes only make up about 1.5 percent of the genome. Researchers have recently shifted their focus to the areas of the genome between the genes, because these regions are now known to contain many functional elements, including regulatory elements.
For many years, researchers assumed that regulatory elements control genes that were physically nearby on the chromosome, but now they know that regulatory elements can control genes that are located far away or even on another chromosome. IDEAS helps find the links between regulatory elements and the genes they regulate. Understanding these links is critical when considering mutations that may be associated with disease.
“Ninety percent of the mutations identified by GWAS occur in regions that do not code for protein,” said Zhang. “One hypothesis is that these mutations disrupt regulatory elements. If the mutation changes how gene expression is regulated, it can indirectly affect the gene and impact disease risk.”
IDEAS currently incorporates information from a core set of five epigenetic marks in 127 different cell types, gathered from the National Institutes of Health Roadmap Epigenomics Project, and analyzes them simultaneously. Because many diseases do not target every cell type or tissue, this gives the model power to detect which regions or mutations are most relevant to a particular disease—for example, by observing which regulatory regions are “turned on” in certain cell types. Zhang anticipates expanding the number of cell types as data using additional epigenetic marks become available for other cell types.
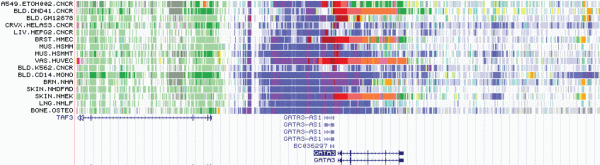
Synthesizing the data
Ultimately, IDEAS helps identify where potential disease-causing mutations occur, in what cell types they occur, and what genes they impact. To do so, it first looks at epigenetic marks across all the cell types and groups similar cell types together. Regulation of genes is likely similar in related cell types; for example, expression of a certain protein might be ramped up in red and white blood cells but reduced or turned off in a skin cell. By assuming that the regulatory regime is similar in grouped cell types, the model is able to borrow information across related cell types.
IDEAS then identifies segments of the genome that share patterns of epigenetic marks and notes their position. Noting similarity in the position of functional elements across cell types improves the model’s ability to accurately break apart relevant segments of the genome. Certain patterns of epigenetic marks are known to be markers for regulatory elements, and these regions are recorded.
Then, the model summarizes all the data by assigning epigenetic states to segments of the genome for each cell type. This process helps condense the complicated epigenetic architecture of the different cell types and suggests how different cell types are regulated.
Once these states are identified, the researcher using IDEAS adds in any information that is already known about their function and location, a process called annotation. This information is usually pulled from public databases where scientists share information about the known functions of some genetic sequences.
“The model only identifies that something important is in each epigenetic state,” said Zhang. “We still have to go in and determine what it is. Even when we already know the function of certain segments, IDEAS can give us new information that could be helpful in understanding its connection to a disease. For example, a lot of the basic functions show up consistently, but we didn’t know if they were connected to certain cell types. When a function appears in only certain cell types, it suggests that function is particular to those cell types.”
In many cases, the function of identified segments may not yet be known. “We rely on the experimentalists to justify what they are,” said Zhang. “But this kind of investigation has been limited because we are overwhelmed by what we can interpret.”
Putting IDEAS to the test
Zhang’s big-data method provides a new way to look at the thousands of experimental datasets generated by GWAS and other genetic studies that are already available in the public domain. IDEAS can help boil down this information into potentially influential regions with relevant annotations. These annotations about segment location and function— and about connections between regulatory elements and genes in various cell types—may help turn the thousands of mutations associated with disease risk into a manageable number of potential targets for drug intervention. Zhang has produced such a list for inflammatory bowel disease, obesity, and bipolar disorder, which could guide the next wave of research and hopefully facilitate drug discovery for these complex diseases.
By compiling annotated genome information generated from IDEAS, it may also be possible for some researchers to benefit without actually running the model themselves. Zhang and his colleagues are working with the Penn State Eberly College of Science Office for Innovation and the Penn State Office of Technology Management to build a web server that will allow other scientists to access this important information. Scientists will be able to upload genetic data they have collected and learn about the regulatory elements and potentially influential mutations within those sequences.
“Unlike the other models currently available, if these scientists have incomplete data—perhaps due to a small budget or narrow focus of interest— IDEAS can help fill in the gaps to produce usable information about the relevant genes and tissues,” said Zhang. “Even if they have a new cell type with just one or two epigenetic marks, it can be annotated and benefit by the full spectrum of information that we have already provided.”
Statistical approaches like IDEAS have allowed researchers to start to make sense of the huge amounts of genetic data that have been produced in the last twenty years, moving beyond just the ABCs of the genetic code. These approaches allow them to pinpoint critical words in genetic sentences and to glean meaning from their order. As researchers come to better understand these sentences and how typos cause disease, the prospects of personalized medicine are even more promising. In time, a patient’s genetic sequence may be an integral part of their medical chart, pointing to an individualized menu of treatment options. Thanks to innovations in a wide variety of scientific disciplines, including statistics, the path to personalized medicine—albeit a long one—may soon start to unfold.