C. R. and Bhargavi Rao Prize | May 16th 2023
David Siegmund will receive the 2023 Rao Prize
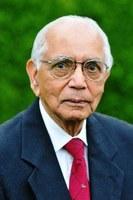
C. R. Rao held the Eberly Chair in Statistics at Penn State University from 1988 – 2001. He now serves as Holder Emeritus of the Eberly Chair in statistics. He was the founding Director of the Center for Multivariate Analysis. A President's National Medal of Science Laureate, Dr. Rao is recognized worldwide as one of the pioneers of modern statistical theory, with multifaceted distinctions as a mathematician, researcher, scientist, and teacher. His pioneering contributions to mathematics and statistical theory and applications have become part of undergraduate and graduate courses in statistics, econometrics, electrical engineering, and many other disciplines at most universities worldwide. In July, Rao will receive the 2023 International Prize in Statistics, the equivalent to the Nobel Prize in the field, for his monumental work 75 years ago that revolutionized statistical thinking.
The C. G. Khatri Memorial Lectureship and P. R. Krishnaiah Memorial Lectureship, which began as two Visiting Scholars programs in 1992, honor the memory of C. G. Khatri and P. R. Krishnaiah by inviting outstanding researchers in statistics to deliver lectures at Penn State.
Image
![]() |
Rao Prize Winner
Stanford University Bio David O. Siegmund is the John D. and Sigrid Banks Professor of Statistics, Stanford University. He received his PhD from Columbia University in 1966, under the supervision of Herbert Robbins. His research contributions have been highly influential and wide-ranging, covering probability theory, sequential analysis, changepoint analysis, and statistical genetics. Prof. Siegmund has held faculty positions at Columbia and Stanford, as well as visiting positions at Hebrew University, the University of Zurich, the University of Heidelberg, Oxford University, Cambridge University, the National University of Singapore, and Free University of Amsterdam. He is the recipient of numerous honors and awards, including election to the National Academy of Sciences, and several named IMS Lectureships. He has authored over 100 papers and authored or co-authored three books. He has a long history of service to the profession. Detection and Estimation of Jumps, Bumps, and Kinks Abstract We consider the problem of segmentation of (usually normal) observations according to changes in their mean. Changes can occur continuously, e.g., a change in the slope of a regression line, or discontinuously, e.g., a jump in the level of a process. Theoretical results will be illustrated by applications to copy number changes, historical weather records, and COVID-19 daily incidence, wastewater analysis, and excess deaths. Sequential detection, confidence regions for the change-points, and difficulties associated with dependent observations will also be discussed. Aspects of this research involve collaboration with Fang Xiao, Li Jian, Liu Yi, Nancy Zhang, Benjamin Yakir, Keith Worsley, and Li (Charlie) Xia. |
Image
![]() |
Signal recovery in single cell data integration Abstract Data integration to align cells across batches has become a cornerstone of most single cell analysis pipelines, critically affecting downstream analyses. Yet, how much signal is erased from data during integration? Currently, there are no guidelines for when biological signals are separable from batch effects, and thus, studies usually take a black-box, trial-and-error attitude towards data integration. I will show evidence that current paradigms for single cell data integration are unnecessarily aggressive, removing biologically meaningful variation. To remedy this, I will present a novel statistical model and computationally scalable algorithm, CellANOVA, to recover biological signal that is lost during single cell data integration. CellANOVA utilizes a “pool-of-controls” design concept, applicable across diverse settings, to separate unwanted variation from biological variation of interest. When applied with existing integration methods, CellANOVA allows the preservation of subtle biological signals and substantially corrects the data distortion introduced by integration. Further, CellANOVA explicitly estimates cell- and gene-specific batch effect terms which can be used to identify the cell types and pathways exhibiting the largest batch variations, providing clarity as to which biological signals can be recovered. This is joint work with Zhaojun Zhang and Zongming Ma. |
Image
![]() |
Khatri Lecturer The value of family data in genetic studies Abstract Early attempts to identify genes causing or increasing susceptibility to diseases relied heavily on family data with multiple affected members. The advent of genotyping arrays, with hundreds of thousands of genetic variants available to query for association with diseases, shifted the focus of genetic studies to (mostly) unrelated participants. These designs ignored information available on disease status from relatives who may not have been enrolled in the study. In this talk, we propose and compare innovative approaches to incorporate familial disease information into genetic association analysis. These approaches provide cost-effective ways to improve statistical evidence and overcome limitations in study designs with insufficient cases or missing genotype information. Incorporating available family history in an analysis of all-cause dementia and hypertension using the exome sequencing data from the UK Biobank resulted in improved significance for known regions. This is joint work with Han Chen and Yanbing Wang. |
Image
![]() |
Speaker Yale University A polynomial time solution to the best subset selection in linear regression Abstract Best subset selection aims to find a small subset of predictors so that the resulting linear model is expected to have the most desirable prediction accuracy. It is not only important and imperative in regression analysis, but also has far reaching applications in every facet of research including computer science and medicine. I will introduce a polynomial algorithm which, under mild conditions, solves the problem. This algorithm exploits the idea of sequencing and splicing to reach a stable solution in finite steps when the sparsity level of the model is fixed but unknown. An information criterion will be defined to guide the algorithm in selecting the true sparsity level with a high probability. I will demonstrate that the algorithm produces a stable optimal solution with probability one, as well as the power of the algorithm in applications. This is a joint work with Junxian Zhu, Canhong Wen, Jin Zhu, and Xueqin Wang. |
Image
![]() |
Speaker George Mason University Semi-parametric learning for explainable models -- a triathlon meets conformal prediction Abstract Determining an explainable model is critical for finding mitigation strategies and understanding reproductive success at high altitudes. In addition to data collection and curation, such model determination depends on the selection of features, variable transformations, and model types, much like a triathlon. This talk presents our semi-parametric learning pipeline for explainable models, which can handle many features or variables and incorporate a change-point component model. We introduce the concept of a transformation necessity-sufficiency guarantee and provide our learning procedure for explainable models, aided by prediction accuracy, stability, and conformal inference. We illustrate the performance of our learning procedure and demonstrate its application in understanding social, physiological, and genetic contributions to the reproductive success of Tibetan women. This is joint work with Shenghao Ye, Cynthia Beall, and Mary Meyer. |
Image
![]() |
Speaker The Hebrew University Detecting DMRs in aDNA Abstract DNA methylation is the addition of a methyl group to a cytosine residue, usually in a CpG context. DNA methylation is an epigenetic mechanism used by cells to control gene expression. The standard technique for determining the methylation profile in the genome involves a bisulfite treatment of the extracted DNA and sequencing. The treatment causes selective deamination of the cytosines, depending on the methylation status, which is expressed in the sequencing output. Unfortunately, the standard technique does not work in ancient DNA (aDNA). The quality and quantity of DNA extracted from excavated skeletons is too low to survive the harsh bisulfite treatment. In the first part of this talk we will describe a bioinformatic technique that enables, in some cases, the reconstruction of the methylome from ancient samples. We will also describe a promising new technique, an alternative to bisulfite treatment, that is being developed for measuring the methylome in aDNA. The second part of the talk will be devoted to the description of statistical tools for the detection of interesting Differentially Methylated Regions (DMRs) in methylomes obtained from aDNA. Applications will be considered, both in the study of human evolution and in the study of life conditions of people in historical and prehistorical past. |
Image
![]() |
Speaker Penn State University Assessing the influence of operational factors on reproducibility of high-throughput biological experiments Abstract High-throughput biological experiments play a crucial role in identifying biologically interesting candidates in large-scale omics studies. The reliability of these experiments depends heavily on the operational factors chosen during their procedures, such as sequencing depth. Therefore, understanding how these operational factors influence the reproducibility of experimental outcomes is critical for designing dependable high-throughput workflows and selecting the most suitable experimental parameters. In this talk, I will introduce a novel framework called correspondence curve regression, which uses a cumulative link model to evaluate how operational factors impact the reproducibility of high-throughput experiments. Unlike commonly used graphical approaches, this framework enables researchers to succinctly characterize the simultaneous and independent effects of covariates on reproducibility and to compare reproducibility while controlling for potential confounding variables. I will also present two extensions to this framework to handle complexities in high-throughput data. The first extension is a segmented regression model, which can identify how operational factors affect the outcomes differently for strong and weak candidates in the experiments. This provides more precise guidance for experiments targeting different types of candidates, enabling researchers to optimize their experimental design for maximum efficiency and reproducibility. The second extension is a method that can handle large amounts of missing data and ensure reliable results. Using this framework, we investigated an important design question for sequencing experiments: How many reads should one sequence to obtain reliable results in a cost-effective way? Our results provide new insights and help biologists determine the most cost-effective sequencing depth to achieve sufficient reproducibility for their study goals. |
If you are planning to attend the light breakfast or lunch, please RSVP here. Registration is not required to attend the lectures.
Schedule of events (May 16th, 2023)
8:30 a.m. - 9:00 a.m. | Registration (Light Breakfast, Tea/Coffee) |
9:00 a.m. - 9:10 a.m. |
Welcoming Remarks by Neeli Bendapudi, Penn State University President |
9:10 a.m. - 9:20 a.m. |
Introduction and Award Presentation by Murali Haran, Statistics Department Head |
9:20 a.m. - 10:20 a.m. |
2023 Rao Prize Recipient Stanford University |
10:20 a.m. - 10:40 a.m. |
Break |
10:40 a.m. – 11:20 a.m. |
2023 Khatri Lecturer Josée Dupuis McGill University |
11:20 a.m. – 12:00 p.m. |
Heping Zhang Yale University |
12:00 p.m. – 2:00 p.m. |
Lunch and Poster Session Huck Life Sciences Building Third floor bridge |
2:00 p.m. – 2:40 p.m. |
Jiayang Sun George Mason University |
2:40 p.m. – 3:20 p.m. |
Qunhua Li Penn State University |
3:20 p.m. – 3:40 p.m. |
Break |
3:40 p.m. – 4:20 p.m. |
Benjamin Yakir The Hebrew University |
4:20 p.m. – 5:00 p.m. |
2023 Krishnaiah Lecturer Nancy Zhang University of Pennsylvania |
5:00 p.m. – 5:10 p.m. |
Concluding Remarks Murali Haran |
Location
The one-day (8:00 am - 5:00 pm) conference is held on the University Park campus of Penn State University in 100 Huck Life Sciences Building, Berg Auditorium. University Park campus map for more details.
Poster Session Information
All Rao Prize Conference registrants, in particular statistics Ph.D. students, are invited to participate in the poster session. The deadline for abstracts is Tuesday, April 25th. Lunch will be provided.
Where: Third floor bridge of the Huck Life Sciences Building
When: 12:00 pm - 2:00 pm
Organizing Committee Contacts
Nicole Lazar (Chair), Lingzhou Xue, Ephraim Hanks, Yubai Yuan
Co-sponsored by
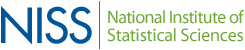