Lindsay Visiting Assistant Professor Program
The Lindsay Visiting Assistant Professor Program was created in 2015 to honor Professor Bruce Lindsay, a leading statistician, mentor and a long-time faculty member of Penn State Statistics.
Current Lindsay Assistant Professors
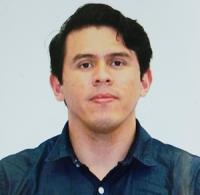
Carlos J. Soto
Carlos is a visiting assistant professor at Penn State.
Soto received his Ph.D. in Biostatistics from Florida State University in 2020. He received his MS in Mathematics from University of Wisconsin - Milwaukee in 2015, and an AB in Mathematics from Ripon College in 2011.
He joined Penn State as a visiting assistant professor in 2020.
Former Lindsay Assistant Professors
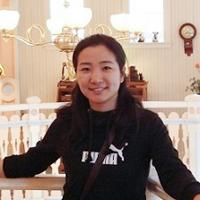
Danning Li
Danning received her Ph.D. in 2013 in Statistics from the University of Minnesota, Minneapolis, advised by Professor Tiefeng Jiang. From 2013-2015, she was a post-doctoral research associate under the supervision of Professor Richard Samworth at the University of Cambridge, England.
Li worked subsequently as an Assistant Professor of Statistics at Jilin University, China and then in 2017 she joined the Department of Statistics at Penn State as a Lindsay Visiting Assistant Professor.
Li’s research areas include random matrix theory, high-dimensional statistical inference, empirical process, and applications to biological science, network science, and social science. Her papers have been published in journals in probability and statistics, including the Journal of Theoretical Probability, the Journal of Mathematical Physics, the Institute of Electrical and Electronics Engineers (IEEE) Transactions on Information Theory, and Statistica Sinica.
Li’s publications include results on the spectra of truncated random unitary matrices; these results are germane to applications involving quantum systems with absorbing boundaries, optical and semiconductor superlattices, quantum conductance, and the distribution of resonances for open quantum maps. She has also published research on the smallest eigenvalues of random matrices, a problem which arises in electrical engineering and in multivariate statistical analysis; her research connects the limiting distributions of the smallest eigenvalues with the celebrated Tracy-Widom probability distributions. Most recently, Li has obtained results that connect Stein’s method of unbiased risk estimation with the estimation of high-dimensional covariance matrices.
In current research, Li is now working with her co-authors to develop power enhancement tests for high-dimensional means, covariance matrices, regression models, and related network models.
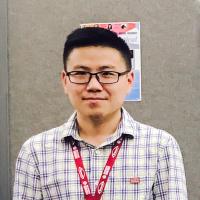
Zhou Lan
Zhou Lan joined the Department of Statistics at Penn State University as a Lindsay Visiting Assistant Professor in 2019. Before joining Penn State Statistics, he obtained a Ph.D. degree in Statistics in the Department of Statistics at North Carolina State University and a Master of Science in Statistics in the School of Mathematics at Georgia Institute of Technology. He is the winner of North Carolina State University Paige Plagge Award. This award is given for good citizenship to "a graduate student with an outstanding academic record, who in the judgment of the committee has especially enhanced the life of fellow students with encouragement, generosity and/or humor."
Zhou’s primary research interests are in the areas of Bayesian nonparametrics, Bayesian methods, spatial statistics, spatial random fields, and neuroimaging. His major contribution is "spatial modeling of positive definite matrices, with applications to diffusion tensor imaging (DTI)". DTI is a popular neuroimaging tool in revealing the brain’s tissue structure. DTI maps and characterizes the 3-D diffusion of water molecules as a function of the spatial location. The diffusion process in the brain reflects interactions with many obstacles, such as fibers, thereby revealing microscopic details about the underlying tissue architecture. Unlike ordinary images where scalars are summarized for each voxel, a distinguishing feature of DTI is each voxel is associated with a 3 × 3 symmetric positive definite matrices. Spatial statistical analysis of DTI data is challenging due to the difficulty of modeling positive matrix-variate responses. We proposed two approaches: a spatial Wishart process (a continuous random field) and a Potts model (a discrete random field). Both are spatial random fields supporting (inverse) Wishart matrices. The associated papers won the 2019 ICSA Applied Statistics Symposium Student Paper Competition and 2019 ASA Student Paper Competition.
At Penn State Statistics, he will be continuing working on spatial statistics, epidemiology, disease mapping, and statistical inferences of positive definite matrices.
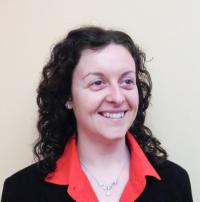
Marzia Cremona
Cremona joined the Department of Statistics at Penn State as a post-doctoral researcher in 2016, working with Francesca Chiaromonte and Kateryna D. Makova, and in 2017 she was appointed to a Lindsay Visiting Assistant Professorship. Marzia Cremona received her B.Sc. in 2009, and M.Sc. in 2011, in Mathematics from the Università degli Studi di Milano, Italy. She obtained in 2016 her Ph.D. in Mathematical Models and Methods in Engineering from the Politecnico di Milano, with a thesis entitled "Statistical methods for omics Data." Her doctoral research advisor was Prof. Piercesare Secchi with co-advisors Prof. Laura M. Sangalli and Prof. Simone Vantini.
Cremona’s primary research interests are in the areas of statistical learning, computational statistics, and statistical “omics”. In her research, Cremona develops statistical and computational methods for the analysis of large, high-dimensional, and complex data – in particular, functional data. An important aspect of her research is its collaborative and multidisciplinary nature; indeed, much of her work is at the interface of statistics and computational biology, and her main application areas are the biomedical and “omics” sciences.
Among her publications are articles on the clustering of ChIP-seq data using peak shape or shape indices, the genome-wide effects of non-B DNA on polymerization speed and error rate, and the influence of the genomic landscape on the integration and fixation of endogenous retroviruses. In research in other areas, she has also studied methods for predicting railway wheel wear using kriging methods.
In research that was completed recently, Cremona has announced results on high-resolution views of adaptive events and on probabilistic methods for clustering and motif discovery in functional data.
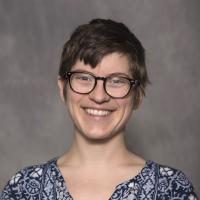
Daisy Philtron
Philtron was a Lindsay Assistant Professor from fall 2015 to spring 2017. She obtained her Ph.D. in statistics from Penn State in 2014. Her research interests focus on statistical approaches to the analysis of biological and genetic data and the pedagogy of teaching statistics.
As a Lindsay Fellow Philtron worked with Dr. Ben Shaby on a project combining diverse data types into a single integrated analysis. They developed a Bayesian three-groups model to classify genetic targets as belonging to one of three groups: beneficial, deleterious, or null. The most current application of this work includes the joint analysis of both microarray and SNP data to investigate possible genetic contributors to Parkinson's disease.
Philtron also led an effort to revitalize Penn State's largest statistics course. During her time as a Lindsay Fellow Philtron planned and implemented an effort to change Stat 200, an intro course with an enrollment of nearly 2000 each semester, from a traditional curriculum to a simulation-based curriculum. She taught the pilot course, developed materials for subsequent semesters, and worked with a team of faculty to coordinate all sections of the class. After the conclusion of her Lindsay professorship, Philtron joined the Penn State statistics faculty as an Assistant Research Professor, where she is continuing her work with Dr. Shaby and developing further course material for the undergraduate program. In 2018 Philtron and Dr. Shaby won a 5-year NSF grant to support their continued research.