New Challenges for Big Data Clustering
Clustering is a fundamental machine learning technique.
Statistics Professor Jai Li says besides high dimensions and large volume, a big data environment also poses the challenge of integrating clustering results at distributed sites, a problem called multi-source clustering. In this project, Li and her team develop novel probabilistic graph models with latent structures and optimization methods for clustering big data. Applications to bioinformatics, image analysis, and trustworthy machine learning will be explored.
Clustering is a major paradigm for unsupervised learning. In scientific exploration, clustering is often the first stage analysis to discover patterns in data, the results of which motivate researchers to make hypotheses for the next in-depth investigation. A byproduct of statistical-model-based clustering is a density estimator. Clustering is also a crucial concept for interpretable machine learning algorithms.
Li and her research team work at the forefront of machine learning techniques and develop data analysis tools for a broad range of scientific and engineering domains. Clustering and density estimation are fundamental problems in statistics. Li and her team are advancing methodologies to address challenges raised by the current big and complex data.
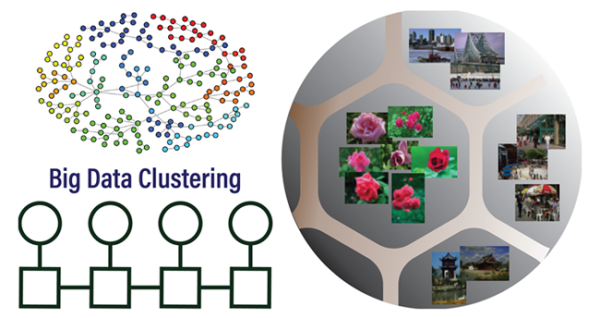
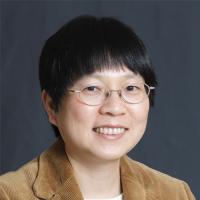
Jai Li is a Professor of Statistics and Computer Science at Penn State. Her current research work includes high dimensional clustering, learning in the Wasserstein metric space, hidden Markov models, and applications in biomedicine, computational psychology, and meteorology.