Detecting Disease Outbreaks with Traveler Data
Statistics Associate Professor Le Bao and colleagues Ying Zhang and Xiaoyue Niu have published a paper titled, What Can We Learn from the Travelers Data in Detecting Disease Outbreaks -- A Case Study of the COVID-19 Epidemic., discussing how traveler case reports could aid in a timely detection of a disease outbreak.
Travel is a potent force in the emergence of disease. Le Bao and colleagues discussed how the traveler case reports could aid in a timely detection of a disease outbreak. Using the traveler data, they estimated a few indicators of the epidemic that affected decision making and policy, including the exponential growth rate, the doubling time, and the probability of severe cases exceeding the hospital capacity, in the initial phase of the COVID-19 epidemic in multiple countries. They imputed the arrival dates when they were missing. We compared the estimates from the traveler data to the ones from domes-tic data. Le Bao and colleagues quantitatively evaluated the influence of each case report and knowing the arrival date on the estimation, and developed a simple disease detection criterion that could help make future decisions.
Using only the travel report data, they estimated the travel origin’s daily exponential growth rate in a moving window fashion that mimic the reality, and examined the date from which the growth rate was consistently above 0.1 (equivalent to doubling time<7 days). Le Bao and his colleagues found those dates were very close to the dates that critical decisions were made such as city lock-downs and national emergency announcement.
In addition, their estimated probability of severe cases exceeding the hospital capacity hit above 0.9 on the day Wuhan announced lock-down. Using only the traveler data, if the assumed epidemic start date was relatively accurate and the traveler sample was representative of the general population, the growth rate estimated from the traveler data was consistent with the domestic data. They also discussed situations that the traveler data could lead to biased estimates.
From the data influence study, they found more recent travel cases had a larger influence on each day’s estimate, and the influence of each case report got smaller as more cases became available. Le Bao and his colleagues provided the minimum number of exported cases needed to determine whether the local epidemic growth rate was above a certain level, and developed a user-friendly Shiny App to accommodate various scenarios.
The traveler data are useful information that help the early detection of a disease outbreak in the travel origin. The traveler data also have limitations that would need further information to refine. Le Bao and his colleagues advocate that countries should work in a collaborative way to share the traveler information about the travel dates and more detailed travel history at sub-national level, in a timely manner.
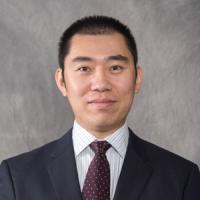
Le Bao is an Associate Professor of Statistics at Penn State. His research focuses on using statistical models to address global health issues such as HIV epidemics.