Moving to a World Beyond “p < 0.05”
How do scientists determine whether or not results are interesting, important, worth pursuing further? In part, this has to do with statistical analysis of the data collected. This project looks at one of the traditional aspects -- "statistical significance" and how to move away from it.
Professor of Statistics, Nicole Lazar, co-edited a Special Issue of "The American Statistician" (sort of "Scientific American" for statisticians - a general-interest, less technical journal) on how to move statistical inference into the 21st century, by moving beyond arbitrary thresholds used traditionally for establishing "statistical significance."
Statistics is used to make many decisions in science: efficacy of new cancer treatments, efficacy of treatments for new diseases (such as COVID-19), and many more questions of broad public interest. It's crucial that we make those decisions in a principled, transparent, and (hopefully) replicable way. This project aims to improve the use of statistics when we make these important decisions.
The Special Issue gathered papers from scientists and statisticians with a variety of perspectives, backgrounds, and opinions. Some of these were invited, and others were contributed after a general call to the statistics community for papers. There were articles on different aspects of science, different proposals for how to modernize statistical inference, how to change the way that we educate students. As co-editor of the Issue, Lazar was also co-author on the lead editorial that summarized the 40+ papers.
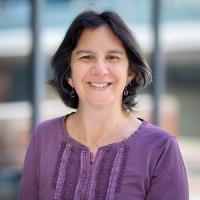
Nicole Lazar is a Professor of Statistics and Director of Online Programs at Penn State. Her research interests include the foundations of statistical inference and the analysis of functional neuroimaging data. In particular, she has worked on fundamental inferential topics such as model selection, multiple testing problems, and likelihood theory, specifically in the context of modern large-scale data analysis problems.