C. R. and Bhargavi Rao Prize
Grace Wahba awarded the 2019 Rao Prize
The C. R. and Bhargavi Rao Prize was established by C. R. and Bhargavi Rao to honor and recognize outstanding and influential innovations in the theory and practice of mathematical statistics, international leadership in directing statistical research, and pioneering contributions by a recognized leader in the field of statistics.
The C. G. Khatri Memorial Lectureship and P. R. Krishnaiah Memorial Lectureship, which began as two Visiting Scholars programs in 1992, honor the memory of C. G. Khatri and P. R. Krishnaiah by inviting outstanding researchers in statistics to deliver lectures at Penn State.
![]() |
Rao Prize Winner "How to incorporate personal densities into predictive Abstract
We are concerned with the use of personal density functions or personal sample densities as subject attributes in prediction and classification models. The situation is particularly interesting when it is desired to combine other attributes with the personal densities in a prediction or classification model. The procedure is (for each subject) to embed their sample density into a Reproducing Kernel Hilbert Space (RKHS), use this embedding to estimate pairwise distances between densities, use Regularized Kernel Estimation (RKE) with the pairwise distances to embed the subject (training) population into an Euclidean space, and use the Euclidean coordinates as attributes in a Smoothing Spline ANOVA (SSANOVA) model. Elementary expository introductions to RKHS, RKE, and SSANOVA occupy most of this talk.
|
![]() |
Khatri Lecturer Abstract The topic of functional time series has received some attention recently. This is timely as many applications involving space-time data can benefit from the functional-data perspective. In this talk, I will start off with the Argo data, which have fascinating features and are highly relevant for climate research. I will then turn to some extensions of stationarity in the context of functional data. The first is to adapt the notion of intrinsic random functions in spatial statistics, due to Matheron, to functional data. Such processes are stationary after suitable differencing, where the resulting stationary covariance is referred to as generalized covariance. A Bochner-type representation of the generalized covariance, as well as preliminary results on inference, will be presented. The second extension considers intrinsic stationarity in a local sense, viewed from the perspective of so-called tangent processes. Motivations of this work can be found from studying the multi-fractional Brownian motion. |
![]() |
Krishnaiah Lecturer "Non-stationary spatial data: think globally act locally" Abstract Large spatial data sets are now ubiquitous in environmental science. Fine spatial sampling or many observations across large domains provides a wealth of information and can often address new scientific questions. The richness and scale of large datasets, however, often reveal heterogeneity in spatial processes that add more complexity to a statistical analysis. Our new approach is to estimate spatially varying covariance parameters in a local manner but then encode these into a sparse Markov random field model for global representation. This strategy makes it possible to estimate and then simulate (unconditional) non-stationary Gaussian processes. This approach is illustrated for the emulation of surface temperature fields from an ensemble of climate model experiments (Community Earth System Model Large Ensemble) and showcases efficient computation using parallel methods and sparse matrices. Current methods in spatial statistics inherit the foundational work in nonparametric regression and splines that was pioneered by Grace Wahba and others. This talk will also trace some of the threads of this research to environmental statistics. |
![]() |
Speaker Ming Yuan Columbia University "Low-Rank Tensor Methods in High Dimensional Data Analysis" Abstract A Large amount of multidimensional data in the form of multilinear arrays, or tensors, arise routinely in modern applications from such diverse fields as chemometrics, genomics, physics, psychology, and signal processing among many others. At the moment, our ability to generate and acquire them has far outpaced our ability to effectively extract useful information from them. There is a clear demand to develop novel statistical methods, efficient computational algorithms, and fundamental mathematical theory to analyze and exploit information in these types of data. In this talk, I will review some of the recent progress and discuss some of the present challenges. |
![]() |
Speaker Bharath K. Sriperumbudur Penn State University "Distribution Regression: Computational vs. Statistical Trade-offs" Abstract Distribution regression is a novel paradigm of regressing vector-valued response on probability measures where the probability measures are not fully observed but only through a finite number (m) of samples drawn from them. This paradigm has many applications in forensics, climate sciences, speaker recognition, etc. In our work, we investigate this paradigm in a risk minimization framework involving reproducing kernel Hilbert spaces and propose a ridge regressor based on kernel mean embeddings. We investigate the computational vs. statistical tradeoff involving the training sample size (N) and the number of samples (m) drawn from each probability measure and show the minimax optimality of the regressor for certain growth behavior of m with respect of N with the growth rate being dependent on the smoothness of the true regressor. |
![]() |
Speaker Alex Smola Amazon Web Services "From features to kernels on graphs and back again" Abstract In this talk I will review statistical models for learning on graphs. Broadly speaking, they can be divided into function-based and feature-based models. In the former, we attempt to assign values to vertices on graphs directly. Graph Laplacians, differential operators and (diffusion) kernels on graphs fall into this category. They come with good characterizations of the function classes associated with them, albeit at the expense of scalability. To address the latter, in practice one often resorts to feature space based methods which assign attribute vectors to vertices before estimation. I will show how this leads to vertex update functions and deep learning on graphs. Besides discussing a number of different models (stationary and iteration based) I'll cover the challenges of making large scale models practical in a higher level language such as Python and I will discuss the associated API. |
![]() |
Speaker Hao Helen Zhang "Scalable and Model-free Methods for Multiclass Probability Estimation" Abstract Classical approaches for multiclass probability estimation are mostly model-based, such as logistic regression or LDA, by making certain assumptions on the underlying data distribution. We propose a new class of model-free methods to estimate class probabilities based on large-margin classifiers. The method is scalable for high-dimensional data by employing the divide-and-conquer technique, which solves multiple weighted large-margin classifiers and then constructs probability estimates by aggregating multiple classification rules. Without relying on any parametric assumption, the estimates are shown to be consistent asymptotically. Both simulated and real data examples are presented to illustrate the performance of the new procedure. |
Schedule of events
8:30 a.m. - 9:00 a.m. | Registration (Tea/Coffee) |
9:00 a.m. - 9:30 a.m. |
Welcoming Addresses & Award Ceremony |
9:30 a.m. - 10:30 a.m. | 2019 Rao Prize Recipient Grace Wahba |
10:30 a.m. - 10:50 a.m. |
Tea/Coffee Break |
10:50 a.m. – 11:30 a.m. |
Ming Yuan Columbia University |
11:30 a.m. – 12:10 p.m. |
Bharath Sriperumbudur Penn State University |
12:10 p.m. – 2:00 p.m. |
Lunch and Poster Session Life science Third-floor bridge |
2:00 p.m. – 2:50 p.m. |
2019 Khatri Lecturer Tailen Hsing Univ of Michigan |
2:50 p.m. – 3:30 p.m. |
Alex Smola, Amazon Web Services |
3:30 p.m. – 3:50 p.m. |
Tea/Coffee Break |
3:50 p.m. – 4:30 p.m. |
Helen Zhang University of Arizona |
4:30 p.m. – 5:20 p.m. |
2019 Krishnaiah Lecturer Douglas Nychka Colorado School of Mines |
5:20 p.m. – 5:40 p.m. |
Concluding Remarks |
Location
The one-day (8:00 am - 5:00 pm) conference was held on the University Park campus of Penn State University in 100 Life Sciences, Berg Auditorium. University Park campus map for more details.
Poster Session Information
All Rao Prize Conference registrants, in particular statistics Ph.D. students, are invited to participate in the poster session, third-floor bridge of the Life Sciences Building from 12:10 pm - 2:00 pm
* We strongly encourage students in the Statistics Ph.D. program in particular.
Organizing Committee
Lorey Burghard, Sriperumbudur, Bharath, Dennis Lin, Qunhua Li
Co-sponsored by
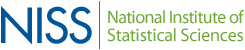
Contact
For further information, please contact Lorey Burghard at lfb109@psu.edu